The team behind Libratus, the poker bot that beat a team of pros for more than $1.7 million, has finally revealed how they created their artificial intelligence (AI) card player.
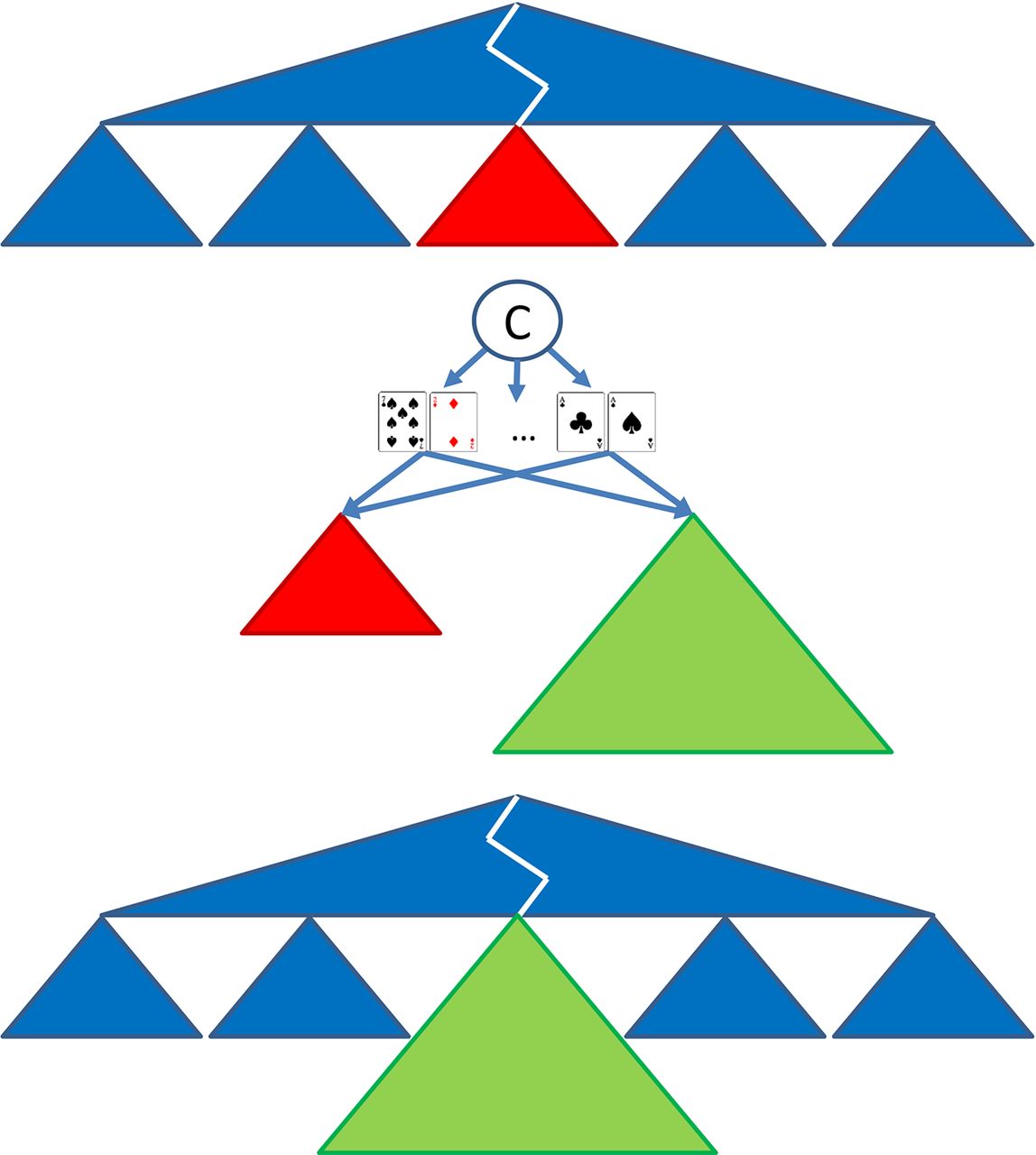
Outlining their raison d’être and subsequent methods in a research paper published in the journal Science, authors Noam Brown and Tuomas Sandholm of Carnegie Mellon University described the mammoth task of creating AI capable of cracking the code of a game as complex as no-limit Texas hold’em.
Cards Against Humanity
For those with short memories, Libratus stuck it to four poker pros back in January 2017. After playing Dong Kim, Jason Les, Bjorn Li, and Doug Polk heads-up for 120,000 hands, the poker bot won by a margin of $1,776,250.
Beyond the convincing victory, the performance did what many assumed was either impossible or, at least, many years away from happening: AI mastery of no-limit hold’em.
Although Libratus hasn’t “solved” the game by any imaginative stretch, the program showed that AI is now capable of beating some of the world’s top pros.
In a paper titled, “Superhuman AI for heads-up no-limit poker: Libratus beats top professionals,” Brown and Sandholm explain the rise of Libratus, and acknowledged tackling the lack of perfect information in the game as the toughest obstacle to overcome.
“Hidden information makes a game far more complex for a number of reasons,” the authors wrote. “Rather than simply search for an optimal sequence of actions, an AI for imperfect-information games must determine how to balance actions appropriately, so that the opponent never finds out too much about the private information the AI has.”
When a lack of direct information about a player’s hand is coupled with unrestricted betting, the number of variables in play becomes extremely vast. This necessitated a three-pronged approach to creating an advanced poker bot capable of competing at a higher level.
Subgame Solutions
First, Libratus was programed with an abstraction of the game to give the bot a basic understanding of general scenarios and game-theory for optimal play. This information formed the basis of the software’s moves in early rounds, when its human opponents didn’t have a read on how it was going to play.
Second, as the game develops, Libratus uses nested subgame solving. This basically takes a more detailed abstraction of subgames (i.e. the specific game in real-time rather than poker in general) and conducts an analysis of what’s going on in the moment. This information is then compared to the general abstractions defined in the first instance.
The major innovation in this area was how Libratus was able to add new information to its calculations to adapt, in real time, for when a player made an unexpected move.
Finally, Libratus was able to self-improve. After taking general and game-specific information, the bot could teach itself and refine its database of knowledge. Another way of looking at it is that Libratus could take information obtained in the first level and rewrite it based on new information and calculations from level two.
While the nuances of Libratus and how it beat four pros are a little more detailed, a key takeaway from the recently published paper is that AI is now hugely advanced, and capable of advancing itself. By having the ability to take general principles and refine them at speed based on new information is the reason why bots are now, in this instance, better poker players than humans.